Microsoft 365. The way it should be.
ShareGate is your true out-of-the-box management solution for your big migrations and your everyday Teams and SharePoint operations.
Easy-to-use, from migration to management
Migration
Migrate to Microsoft 365, merge tenants, move Teams teams, Planner plans and more in a snap. Now with Mailbox Migration!
Provisioning
Create dynamic templates with multiple approvers so end users can create their workspaces with your guardrails in place.
Reporting
Centralized and actionable SharePoint and Teams reporting and monitoring.
Automated governance
Automate everyday IT tasks in Teams and SharePoint.
Permissions management
Centralized and flexible management of permissions in SharePoint and Teams.
Administration
Transform your environment following best practices. Stop fighting fires as the come up, and improve productivity.
Trusted Microsoft 365 management tool for over 75,000 IT professionals worldwide.

Migration
Move what you want where you want it.
ShareGate now supports tenant-to-tenant mailbox migration. Our simple tool is good to go, from SharePoint on-prem or cloud-to-cloud. Migrate to Microsoft 365. Move teams or channels. Migrate mailboxes. High five!
Reporting
Stay in the know. Stay on track.
Our built-in and custom reports give you a total overview of your SharePoint and Teams inventory. See what exists. Who created it. If it’s still alive. And course correct as needed.
Provisioning
Next-generation digital workspace management.
Create dynamic templates that accelerate adoption. IT sets guardrails. Users get purpose-fit workspaces instantly with the “yes” or “no” from multiple approvers. All using the power of SharePoint’s configurations. Boom!
Our clients agree: ShareGate is easy to use and easy to choose
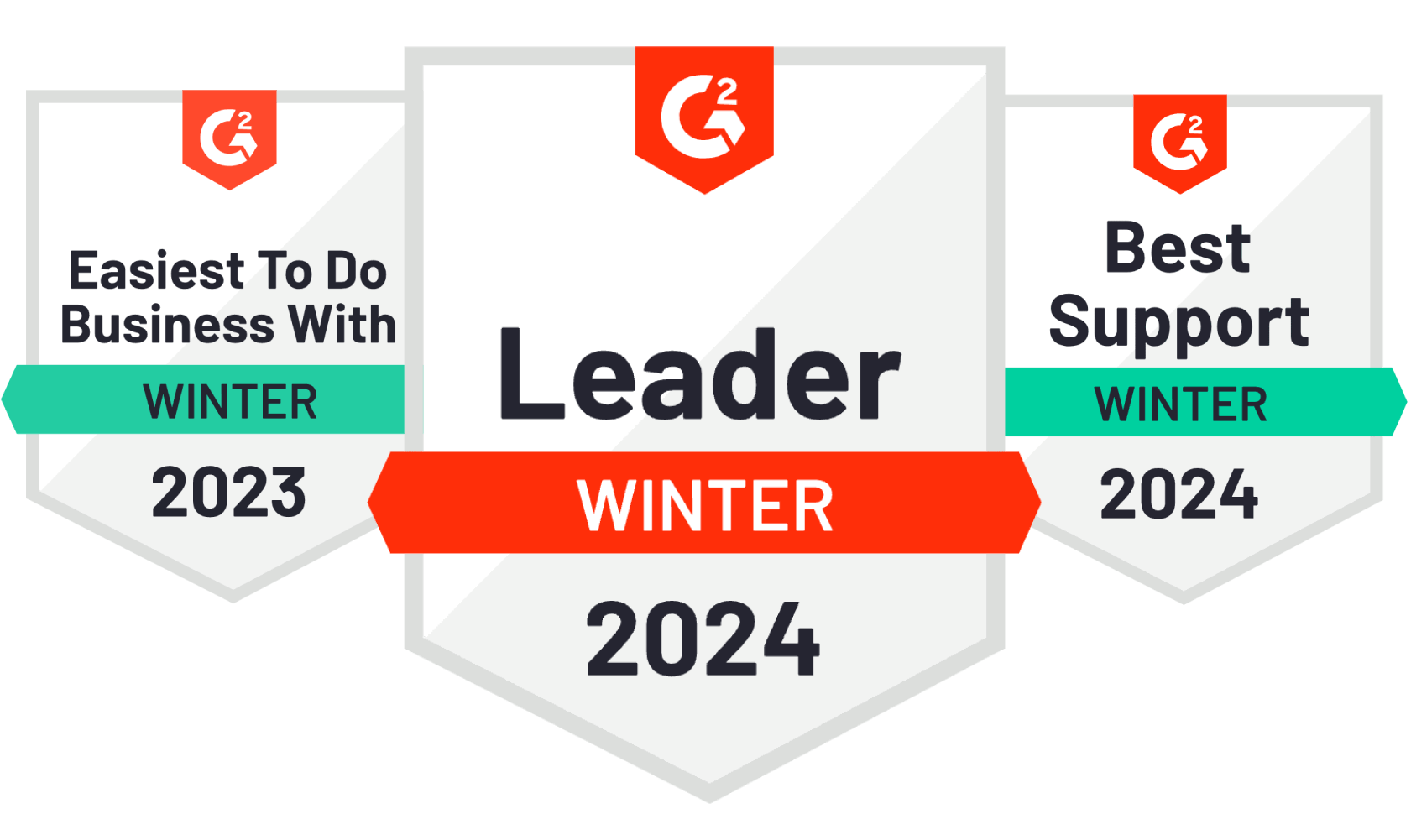
ShareGate has helped us increase IT efficiency and allowed us to put more time where we are needed most. On a smaller migration, ShareGate saves me hours. On our main cloud migration, it gave me weeks back
— Matthew Carter
Cloud Engineer III, IDEMIA Identity and Security North America
ShareGate is very user friendly and really makes my job much easier. We constantly have users requesting IT to copy Teams or SharePoint sites, and without ShareGate, it really is a very lengthy and manual process.
— Jennifer J
Application Engineer, Rocket Central
ShareGate is simple to use and incredibly powerful. I could not have managed our migration projects and ongoing M365 maintenance nearly as effectively as I have without it.
— Alex Burgess
Senior Infrastructure IT Technician, HSDC
We make your biggest Microsoft 365 admin jobs easy.
Skip the scripts.
Forget PowerShell, lighten the load with intuitive UI’s using drag-and-drop functionality.
Get self-serve on your side.
Set up your guardrails in a snap and sail worry-free with Microsoft 365. Rest easy knowing users are on the right course.
Keep up with Microsoft.
Focus your efforts in the right places guided by best practices. Free, regular updates keep you ahead of the game.
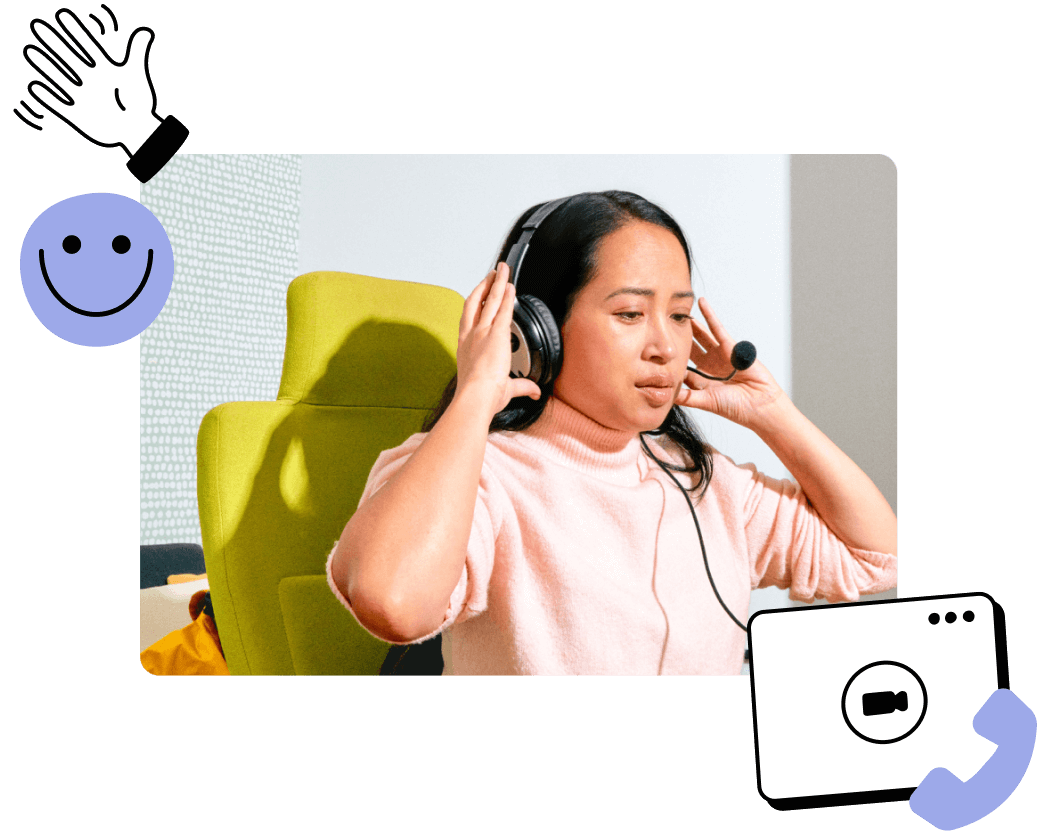
Book a live 1-on-1 demo with our experts
- Hear free advice and insights for your unique use case
- Get a personalized walkthrough of our main features
- Receive a follow-up email with additional resources specific to your needs